AIOps for OT – The Key to Scale AI in Factories - Part I
TL;DR;
Over recent years, AIOps (Artificial Intelligence for IT Operations) has gained attention from companies aiming to enhance operational efficiency and productivity through artificial intelligence (AI).
While initially developed for IT environments, AIOps can also be successfully applied to Operational Technology (OT), where real-time decision-making and low latency are crucial.
In this article, we will explore how AIOps is transforming industrial environments by combining advanced AI techniques with real-time automation.
What is AIOps for OT and Why is It Critical for Industrial Environments?
AIOps was originally designed to optimize IT infrastructure, automating problem detection and allowing systems to self-manage effectively.
In industrial environments, AIOps extends beyond IT, focusing on critical production operations in OT (operational technology environments), encompassing practices from DatOps (data operations) and also MLOps (model operations). The figure bellow tryes to illustrate the overall concept of AIOps with the combination of Data and ML operations.
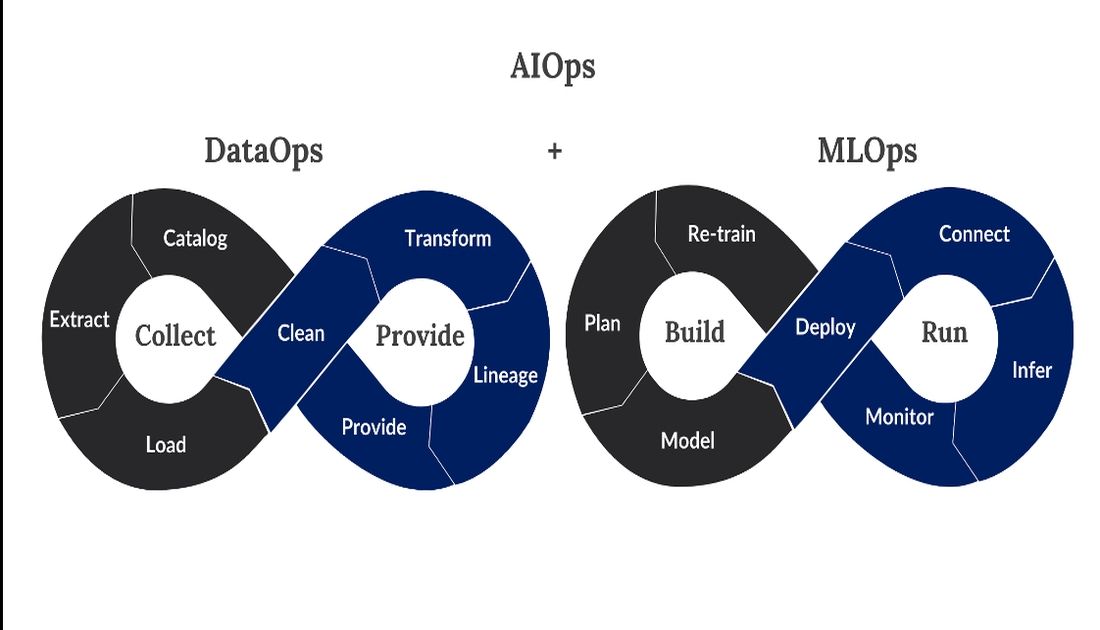
AIOps for OT comes to try to overcome typical challenges to put AI into production in the industrial environment:
• Low Latency: Industrial operations demand near-instantaneous decision-making, especially when AI is working in real-time environments.
• Security: Sectors like mining and manufacturing are highly sensitive to cybersecurity threats, requiring AI solutions to operate securely in air-gapped environments.
• Hardware Efficiency: AIOps optimizes the use of local computing resources, crucial for industries with limited infrastructure or strict on-premises requirements.
The challenges mentioned above arise because OT does not have access to the same infrastructure available in IT environments within large industrial corporations. This is mainly due to the safety and security requirements that industrial equipment must meet, as they operate close to the production process, and any safety or security issue could pose significant risks. In addition to the low latency and security concerns discussed in the previous paragraphs, the OT environment also faces hardware limitations that restrict the availability of cloud technology in OT.
This is why the concept of AIOps makes a lot of sense, as it aims to overcome these technological challenges by applying appropriate concepts and best practices to deploy AI in production despite these limitations.
AIOps in OT: Overcoming Scalability Challenges
One of the biggest challenges in OT is deploying AI models effectively without disrupting operations. Machine learning models in OT environments face obstacles like real-time data demands, strict latency requirements, and the need for secure, disconnected operations.
Beyond the challenges of MLOps, DataOps in the industry faces difficulties such as correctly reading industrial communication systems, like OPC, for example. Typically, these systems provide data to production systems, and we need to perform secure data collection without interrupting the data flow being sent to the production system, while also sending it to the AI system. This means ensuring secure and robust data collection, as well as processing the industrial data, since field sensors can fail, and there is often no measure of the data’s reliability and quality. DataOps addresses these issues by providing reliable data for the MLOps layer, which will use this data for model inference.
Now, with AIOps, data and model governance becomes more structured, enabling:
• Automation of Machine Learning Pipelines: From data collection to model training and deployment, AIOps automates the entire process, reducing human errors and speeding up production timelines.
• Continuous Monitoring: AIOps provides real-time model performance monitoring, allowing for proactive retuning and ensuring models remain accurate over time.
• Scalability: AIOps allows models to be replicated across different production lines or plants with minimal adjustments, making scaling seamless and efficient.
Use Case: Scaling Predictive Maintenance in Manufacturing
In a large-scale manufacturing plant, AIOps was implemented to streamline predictive maintenance. Traditionally, the company relied on manual checks and reactive maintenance, leading to frequent downtimes.
By integrating AIOps, the company automated reliable data collection and processing (DataOps procedures) and also scaled anomaly detection models across several critical pieces of equipment (10) (MLOps procedures), such as conveyor belts. The figure bellow, shows and example of an anticipation of thirteen hours ahead of the unplanned shutdown, the anomaly detection algorithm was already alarm the potential shutdown, which really ocurred.
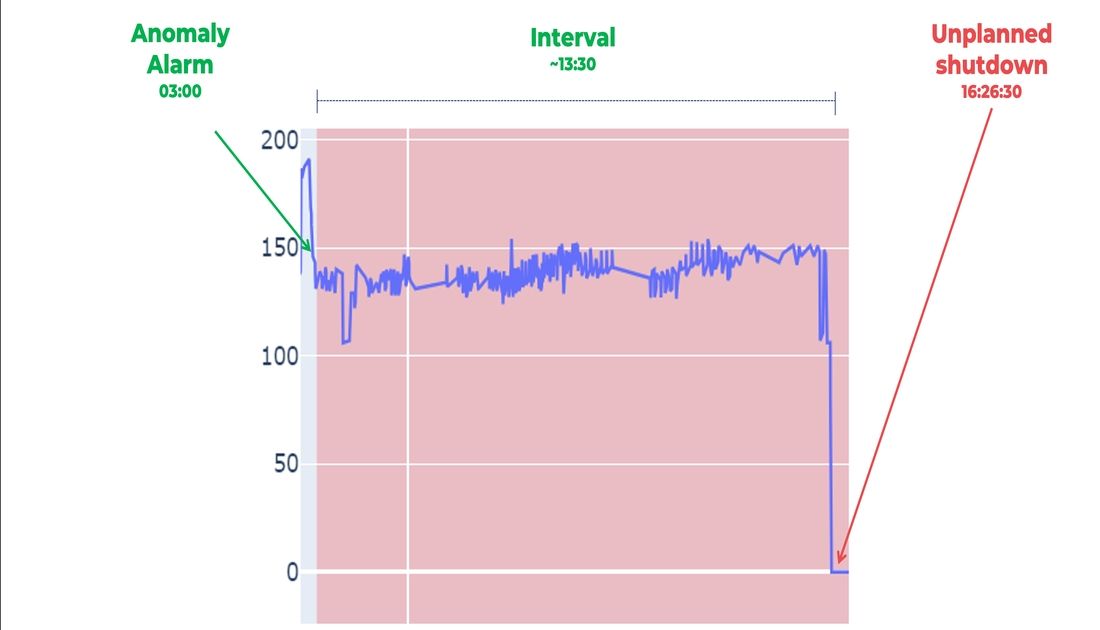
Results:
• Reduced Downtime: Maintenance teams were alerted of potential issues before they occurred, reducing downtime.
• Cost Savings: By automating anomaly detection, the company cut down manual inspection costs.
• Scalability: AIOps enabled the replication of the model across multiple production lines, achieving operational consistency.
Conclusion
AIOps offers a powerful solution to the challenges of AI deployment in OT environments. By optimizing hardware usage, ensuring security, and reducing the time to production, AIOps can transform industrial processes and deliver substantial business value.
What is next?
In the upcoming article, part 2, we will dive deeper into what DataOps and MLOps are, and how all of this makes a lot of sense in helping to scale AI in the industry.