AIOps for OT – The Key to Scale AI in Factories - Part II
TL;DR;
Deploying AI in Operational Technology (OT) environments can revolutionize industrial processes, boosting efficiency and reducing costs.
However, transitioning AI from proof of concept to production in OT presents significant challenges. In this article, we will explore in detail the key obstacles industries face when implementing AI in OT and how AIOps helps overcome them.
Why Deploying AI in OT is More Complex than in IT
Unlike IT environments, which often have flexible connectivity and robust cloud infrastructure, OT environments in industries like mining, manufacturing, and energy operate in real-time, with zero tolerance for delays or failures.
Additionally, there are cybersecurity concerns about exposing the entire set of PLCs (Programmable Logic Controllers) or DCS (Distributed Control Systems) to external networks, even with modern security layers and strategies such as using a DMZ. The following are the major challenges industries face when deploying AI in OT:
1. Low-Latency, Real-Time Operations
Industrial environments require near-instantaneous decision-making. AI models must process and respond to data within milliseconds to prevent costly disruptions. It is also impractical to transmit large amounts of data (such as time series or images) in milliseconds over a standard 10/100 network configuration between OT and IT.
AIOps Solution:
AIOps processes data locally, ensuring models run in real-time without the delays associated with cloud-based solutions. This real-time capability is critical for high-performance industrial operations.
2. Limited Connectivity and Security
Many industrial plants have restricted or no internet access for security reasons. This prevents the use of cloud solutions and increases the difficulty of deploying AI.
AIOps Solution:
AIOps enables on-premises AI edge deployment with full governance over data (DataOps) and models (MLOps), securely managing data and operations in isolated environments. By automating the monitoring of security systems, AIOps efficiently detects vulnerabilities and mitigates risks.
3. Legacy Infrastructure Integration
Many industrial systems run on legacy equipment and protocols that were not designed for modern AI technologies. Although modern options exist, such as OPC UA for Industry 4.0 requirements, most legacy systems in factories still do not use modern communication protocols.
AIOps Solution:
AIOps simplifies integrating AI with legacy systems by using specialized and optimized data connectors and protocols, allowing seamless data collection and machine learning implementation.
4. Scalability and Model Replication
Once an AI model is deployed in one part of a plant, scaling it to other areas without manual intervention becomes a challenge. Additionally, there is a skill gap, as most industrial engineers are not data scientists. This creates an opportunity for no-code and automated machine learning solutions to help bridge this gap.
AIOps Solution:
AIOps combined with no-code user interface and auto-machine learning features, automates model replication across different production lines or sites, allowing teams to scale AI implementations quickly and efficiently without compromising model accuracy.
5. Data Management and Governance
Managing vast amounts of real-time data from industrial sensors and ensuring its quality is a daunting task.
AIOps Solution:
AIOps integrates DataOps practices to ensure high-quality, reliable data management. Automated version control and governance frameworks allow companies to maintain compliance and track model performance over time.
Case Study: Industrial AIOps Applied in a Mining Company in Brazil
A major mining company in Brazil faced challenges deploying AI models in the edge due to limited internet connectivity and a not proper infrastructure/software for AI production, typical in operational technology (OT) environments. The company’s goal was to optimize its production processes while maintaining high security standards and ensuring real-time decision-making capabilities. At the same time, they would like to scale the many use cases that they have experienced before during PoC (proof of concept) projects.
Our AIOps platform (SIENTIA™) was introduced to tackle these challenges, automating the development and deployment of AI models through no-code interfaces and AutoML capabilities. This approach allowed the client’s engineering team, which had no prior experience in data science, to independently scale their AI initiatives.
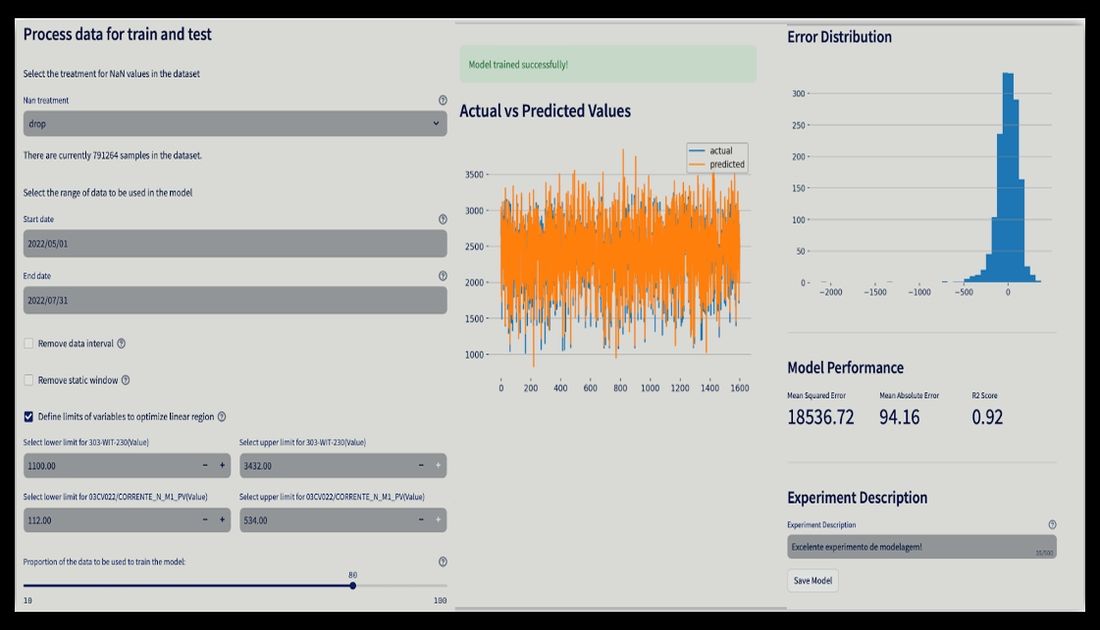
By leveraging AIOps, the mining company developed and scaled over ten AI models, including virtual sensors for quality prediction and anomaly detection models across multiple sites. With integrated MLOps and DataOps frameworks, the company maintained full governance and compliance, ensuring that their models remained accurate and efficient over time.
Results:
• Improved Scalability: The company scaled over 10 models autonomously, thanks to the no-code and AutoML features, reducing dependency on external experts.
• Reduced Deployment Time: The average time from development to deployment for new models was reduced to just one week.
• Increased Production Efficiency: By deploying models across different areas of their operations, the company expects to achieve at least a 0.5% increase in production efficiency, resulting in substantial cost savings.
Use case | Models Developed by Mining Enginering team | Models Developed by third parties | Average time from Development to Production |
Virtual Sensor for Conveyor Belts Scales | 6 | 0 | 1 week/model |
Virtual Sensor for Quality Prediction | 1 | 1 | 2 weeks/model |
Anomaly Detection | 6 | 1 | 1 week/model |
Conclusion
AI deployment in OT environments presents unique challenges, but with AIOps, companies can automate processes, secure operations, and scale their AI efforts effectively. The future of AI in industrial operations depends on overcoming these obstacles, and AIOps is a critical part of that transformation.